The climate change has raised concerns about closing the carbon cycle by converting CO2 and renewable electricity to chemically
stored energy in the form of fuels and commodity chemicals. Among these, long-chain hydrocarbons and alcohols are more attractive
because of their high energy density and value. Recently, reconstructed oxide-derived Cu (OD-Cu) catalysts have shown the potential
to produce multicarbon species at lower overpotentials. However, few simulations have addressed the formation mechanism of these
compounds due to the complex dynamics of this system under high currents, and the exact site for the excellent OD-Cu catalytic
performance remains to be discovered. The DESCRIPTOR project aims to obtain the first generation of CO2 electroreduction catalyst
with useful faradaic efficiencies towards C3+ products by employing computational simulations based on Density Functional Theory
(DFT) and augmented by Machine Learning techniques. Firstly, suitable structures for the OD-Cu materials will be obtained through
large scale Molecular Dynamics simulations based on Machine Learning potentials, by screening the most common ensembles
identified via graph theory. Secondly, the mechanism towards C3+ will be identified via jDFTx scheme, and descriptors of activity and
selectivity will be found through dimensionality reduction techniques. Finally, to assess and compare to experimental work from our
collaborators, the contribution of the solvent/electrolyte and effect of experimental parameters will be investigated via ab initio
Molecular Dynamics and microkinetic modelling. The structures will be characterized via simulations of X-ray Photoelectron
Spectroscopy, and Raman spectra, etc. In summary, the outcome of DESCRIPTOR will have a direct scientific and social impact, by
increasing the basic knowledge on catalysis of achieving renewable fuel sources and improving EU’s industrial competitiveness
within new technologies for CO2 reduction.
DESCRIPTOR
Fellow name: Zan Lian
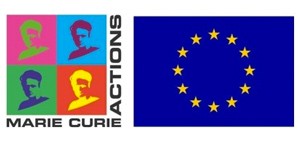
MSCA IF